eftax-lab
Ryosuke Isono
Since my university days, I have participated in eftax internships and have worked on projects for business companies. Currently, I am a member of MDILab, Mathematical Data Informatics (MDI) Laboratory, Department of Computer Science, School of Computing, Tokyo Institute of Technology. My research deals with satellite images obtained by observing the earth’s surface from satellites.
Image sensors on satellites are characterized by three resolutions: spatial, spectral, and temporal.
Sensors with high spatial resolution can capture spatially detailed images, which is useful when you want to know more about spatial shapes, such as the shape of a terrain. Sensors with high spectral resolution can acquire detailed spectral information of light and can identify objects that look the same to humans but are actually different. This makes them useful for analysis to determine where and what substances are contained. Sensors with high temporal resolution can capture the same location with high frequency, making them suitable for capturing images of places that change rapidly over time, such as cities under development.
If you want to analyze satellite imagery in a variety of ways, you want to use a sensor with high resolution for all of them, but in practice this is not the case.
There is a trade-off between the three resolutions, and prioritizing one will reduce the resolution of the others.
Therefore, we are working to estimate all high-resolution image sequences that cannot be captured in reality by combining images captured by multiple sensors with different resolutions. This issue is called STS(Spatio-Temporal-Spectral) fusion, since the images are synthesized at different spatial, temporal, and spectral resolutions.
I am working on this STS fusion using an approach called mathematical optimization, rather than deep learning, which is the current trend. This approach enables highly accurate synthetic even when a large amount of data is not available for learning. In addition, unlike the images we usually take with our smartphones or digital cameras, satellite images observed from satellites are inevitably degraded by various types of noise and data loss due to the principle of observation. However, the method I am developing is designed to be robust against such degradation.
I started my research on STS fusion after entering the master’s program, and one paper was accepted to ICASSP[*1], a flagship international conference in the signal processing area, within the first year of my master’s program. I intend to continue my research on STS fusion for a while, aiming for an even more versatile method that can handle more types of noise and more flexible data for fusion.
Publications
- [*1] Papers presented at ICASSP2023
Ryosuke Isono,Kazuki Naganuma,Shunsuke Ono,「Robust Spatiotemporal Fusion of Satellite Images via Convex Optimization」, IEEE,05 May 2023, DOI: 10.1109/ICASSP49357.2023.10095246
- Papers awaiting peer review submitted to IEEE Transactions on Geoscience and Remote Sensing
Ryosuke Isono, Kazuki Naganuma, Shunsuke Ono, “Robust Spatiotemporal Fusion of Satellite Images: A Constrained Convex Optimization Approach”, Submitted on 1 Aug 2023, https://arxiv.org/abs/2308.00500 *Link to arXiv’s website.
- Personal site for academia Ryosuke Isono
Related
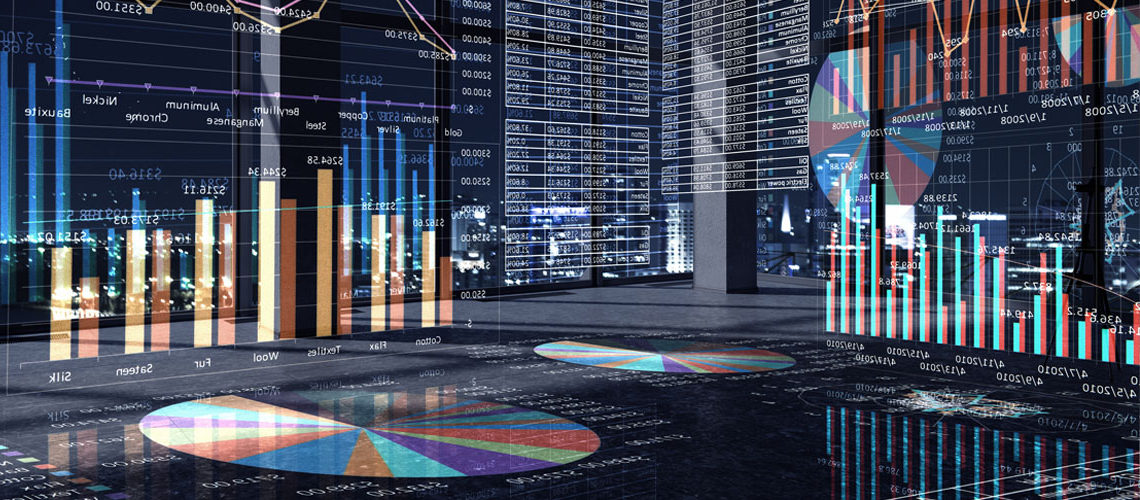
Data Analysis Services
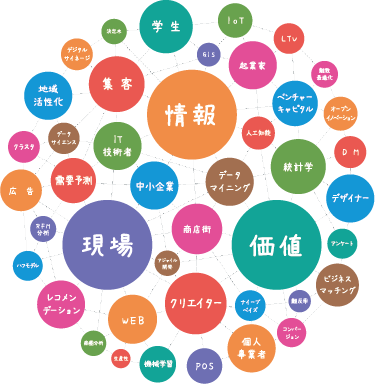